Your cart is currently empty!
Advances in Causal Inference SURD
What it is, why itโs important, and an overview of the existing state of the art
A recent paper on a new, even more refined approach to Causal Inference SURD came to our attention, and we wanted to share a primer on Causal Inference. This is also up on the Prism14 website with links to other Prism14 content on data literacy, people know-how, and materials can-do.
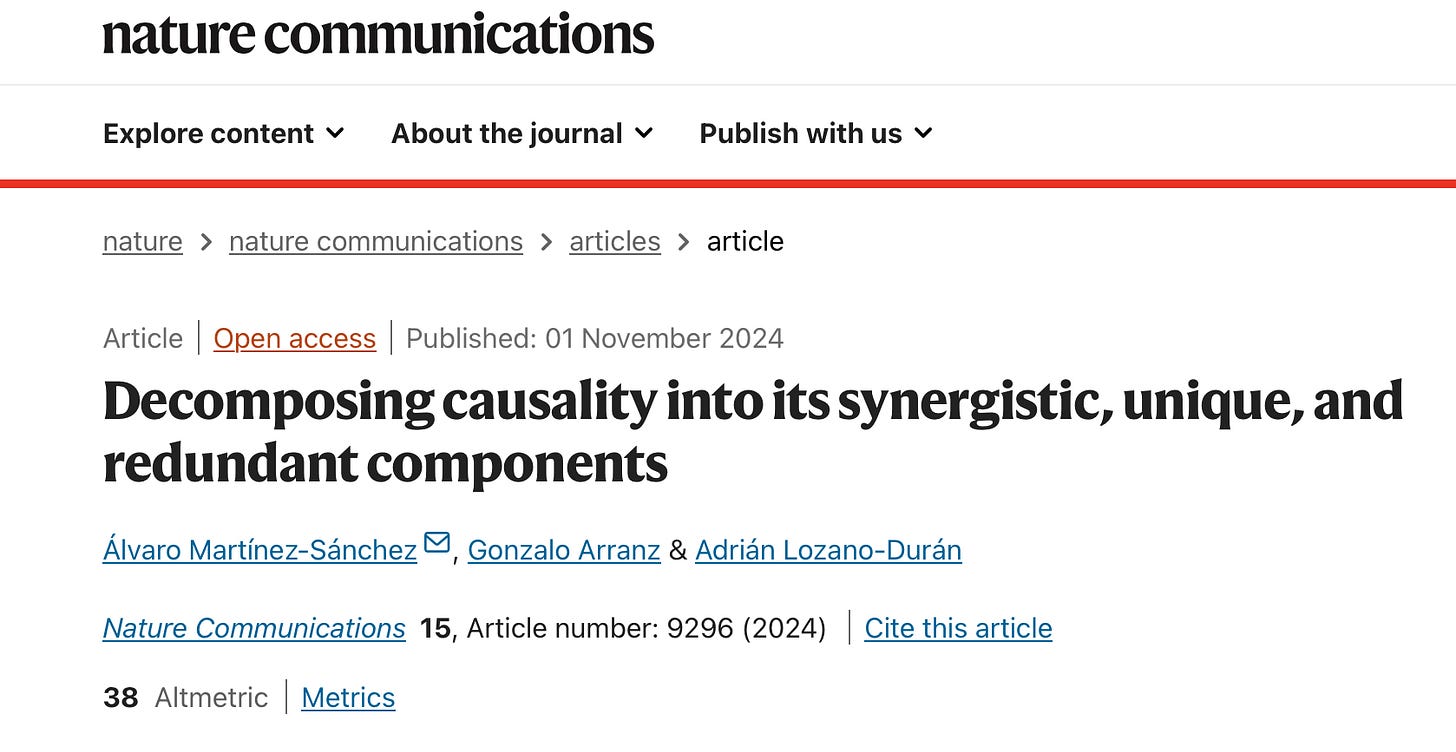
How is Causal Inference Useful?
Causal inference (CI) is useful because it enables researchers and decision-makers to go beyond mere correlation to understand and quantify true cause-and-effect relationships within complex systems.
This knowledge is crucial in numerous fields:
1. Science and Research: CI allows researchers to determine the underlying mechanisms behind observed phenomena. For instance, in epidemiology, CI helps identify risk factors for diseases, and in neuroscience, it is used to uncover relationships between brain activity and behavior.
2. Policy and Public Health: In policy-making, CI helps evaluate the effectiveness of interventions, such as whether a public health campaign reduces disease incidence or if specific policies curb pollution. This is essential for directing resources efficiently toward interventions that yield real benefits.
3. Economics and Social Sciences: In economics, CI methods are used to assess the impact of economic policies (e.g., taxation, subsidies) on outcomes like employment or GDP. In social sciences, CI helps study complex social behaviors and structural inequalities by revealing the causal pathways behind outcomes like educational achievement or income disparities.
4. Artificial Intelligence and Machine Learning: In AI, CI is increasingly used to improve model interpretability and robustness by identifying and integrating causal factors. This is valuable for building AI systems that behave predictably and reliably in real-world settings, where correlation alone may be misleading.
5. Medicine and Clinical Research: CI is critical in clinical research for determining whether a treatment causes a specific health outcome. It enables researchers to distinguish between effective and ineffective treatments, ensuring patient safety and advancing medical science.
6. Environmental and Climate Science: CI helps isolate and understand causal relationships in complex systems like the climate, where human and natural factors interact in ways that are often nonlinear and interconnected. This understanding supports the development of evidence-based climate policies and interventions.
Why is Causal Inference Useful?
Causal inference is essential because it addresses a fundamental limitation of correlation-based analysis: correlation does not imply causation. Without CI, decisions might be based on coincidental associations rather than genuine cause-and-effect relationships, leading to ineffective or even harmful interventions. The following reasons highlight why CI is so valuable:
1. Accurate Decision-Making: CI ensures that interventions and policies are based on evidence of causation, which is crucial for effectiveness and accountability. For instance, in public health, CI clarifies which factors truly reduce disease spread, enabling targeted interventions.
2. Understanding Complex Systems: CI allows scientists and researchers to untangle the complexity of natural and social systems by identifying how different variables influence each other. This insight is invaluable for disciplines like climate science, economics, and social sciences, where variables are often interdependent.
3. Optimizing Resource Allocation: By identifying genuine causal relationships, CI helps organizations allocate resources more effectively. For example, knowing which educational policies causally improve student outcomes enables governments to invest in successful programs while avoiding those with no real impact.
4. Personalization and Precision: CI supports personalized approaches in fields like medicine, where understanding individual responses to treatments can lead to more effective, tailored therapies. It enables the identification of factors that causally affect specific subgroups, refining interventions to meet individual needs.
5. Improving Predictive Models: In fields like AI and machine learning, incorporating causal knowledge into models improves their reliability and robustness. Causal models can better generalize to new data or changes in the environment, making them more useful for real-world applications where correlation-based predictions might fail.
In essence, CI transforms data into actionable knowledge, providing the foundation for informed, evidence-based decisions across a range of fields. Its ability to discern true causal relationships empowers individuals, organizations, and societies to make choices that lead to tangible, positive outcomes.
What Even Is Causal Inference!?
Causal inference is the process of determining whether a relationship between two variables is causalโmeaning that one variable directly influences the otherโrather than simply correlative. Unlike correlation, which merely indicates that two variables move together, causation implies a directional, cause-and-effect relationship. Causal inference methods are central to scientific inquiry as they help distinguish genuine causal relationships from spurious correlations, confounding factors, or coincidental associations. Techniques for causal inference range from randomized controlled experiments to observational methods, including statistical and machine learning approaches. These methods allow scientists to understand complex, interdependent systems by systematically isolating and analyzing the impact of individual variables.
How is Causal Inference Used in Climate Science to Understand the Climate and Weather?
In climate science, causal inference is crucial for understanding complex interactions between various climate drivers (e.g., greenhouse gases, aerosols, land-use changes) and their impacts on global weather and climate patterns. By applying causal inference, researchers can determine the specific drivers behind observed climate trends, such as temperature changes, storm frequency, and precipitation patterns. For instance, causal inference methods allow scientists to isolate the influence of anthropogenic factors like COโ emissions from natural variables like volcanic activity or solar radiation. Methods such as Granger causality, transfer entropy, and model-based approaches are commonly used to infer causation from observational data, given the ethical and practical limitations of experimenting on the climate.
Moreover, causal inference helps identify feedback loops and interactions within the climate system, such as how rising temperatures affect ocean currents, which in turn influence atmospheric circulation patterns. By accurately identifying these causal relationships, climate scientists gain a deeper understanding of the mechanisms driving climate and weather changes, which is essential for improving climate models and predicting future trends.
How is Causal Inference Used in Climate Science to Test Understandings of Appropriate Mitigation Approaches?
Causal inference plays a critical role in testing and validating mitigation strategies in climate science. By analyzing historical and observational data, researchers can evaluate the effectiveness of specific mitigation policies, such as emissions reduction efforts, reforestation projects, or geoengineering techniques. For example, using causal inference, scientists can examine whether policies aimed at reducing emissions have successfully lowered atmospheric COโ concentrations and if these changes have led to measurable impacts on global temperatures or specific weather patterns.
Additionally, causal inference methods enable climate scientists to simulate the potential effects of different mitigation scenarios. By understanding causal relationships, researchers can model how reducing greenhouse gas emissions might affect temperatures, sea levels, and extreme weather events over time. This approach allows scientists and policymakers to test โwhat ifโ scenarios and assess the long-term consequences of various strategies before implementing them. Causal inference thus provides a scientific basis for recommending certain mitigation actions over others, ensuring that resources are allocated to the most effective and sustainable strategies for climate stabilization.
How is Causal Inference Used in Clinical Research?
In clinical research, causal inference is fundamental for determining the effects of treatments, interventions, and exposures on health outcomes. Through randomized controlled trials (RCTs) and observational studies, researchers use causal inference to establish whether a drug or therapy directly impacts patient health. RCTs are often considered the gold standard because they randomly assign participants to treatment or control groups, minimizing confounding factors. However, in cases where RCTs are impractical or unethical, observational causal inference methods, such as propensity score matching, instrumental variables, and mediation analysis, are applied to control for confounders and bias.
Causal inference in clinical research extends beyond determining treatment efficacy to understanding risk factors for diseases, side effects of drugs, and complex interactions within biological systems. For example, causal inference can help identify environmental or lifestyle factors that contribute to chronic illnesses like cancer or heart disease, thus guiding preventive measures and public health policies. Additionally, in personalized medicine, causal inference allows researchers to tailor treatments to individual patient profiles by isolating which factors predict positive outcomes in specific patient subgroups. The insights gained from causal inference are thus crucial for advancing medical science, improving patient care, and optimizing health interventions.
How Causal Inference Fits into Prism14
Weโre discussing causal inference at Prism14 because itโs foundational to understanding and driving meaningful change across complex systemsโa core aspect of our mission. At Prism14, we emphasize skills and insights that empower individuals and organizations to make better-informed decisions, build sustainable systems, and navigate complexity. Hereโs why causal inference (CI) is so relevant for what we do:
1. Empowering Data Literacy: CI is critical to data literacy, one of our central themes. Understanding causal inference helps our readers and students go beyond surface-level insights in data analysis, enabling them to ask deeper questions about โwhyโ and โhowโ rather than just โwhat.โ This aligns with our commitment to improving contemporary skills in data analysis and interpretation, fostering not only technical capability but also a more critical approach to information.
2. Navigating Complexity in Systems and Processes: CI provides tools to disentangle complex, interdependent processesโa major focus of Prism14โs content. Our approach emphasizes continuous improvement and systems thinking, both of which benefit from CI. Whether itโs in sustainability, product development, or small business finance, CI allows us to pinpoint what actions genuinely create positive change and eliminate inefficiencies, thus supporting sustainable and resilient systems.
3. Developing Skills for Real-World Applications: CI principles help in practical, decision-making frameworks for personal finance, business operations, and creative or product developmentโall topics we cover at Prism14. By learning how to distinguish causation from correlation, our readers and students can make smarter decisions, avoid costly mistakes, and apply scientific rigor to their strategies. CI is invaluable for anyone interested in applying data-driven approaches to real-world challenges, which is precisely the audience weโre supporting.
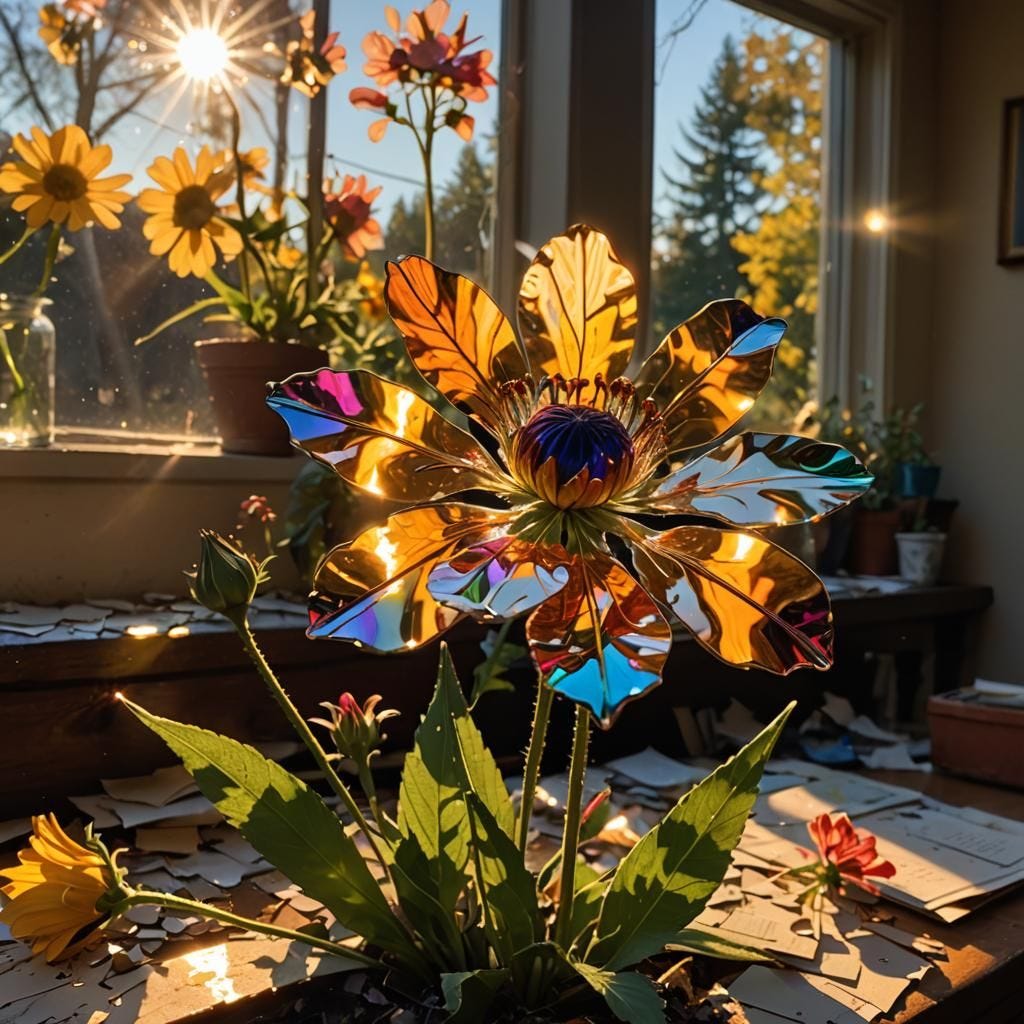
4. Supporting Flow and Engagement in Learning: One of Prism14โs unique focuses is on flow coaching and group dynamics. Causal inference is useful here, as it can shed light on which factors lead to group flow states and engagement. By understanding these causal relationships, we can help people and organizations design environments and processes that maximize productivity, creativity, and satisfaction.
5. Addressing Bias and Building Ethical Systems: CI helps uncover the often-hidden biases and confounders in data, which is essential for creating ethical systems and products. At Prism14, we encourage building systems with integrity and equity. CI provides the tools to critically examine data-driven decisions, ensuring they are not unintentionally perpetuating biases or inequalities.
By integrating causal inference into Prism14โs mission, weโre providing tools and insights that are crucial for anyone who wants to work intelligently with data, foster innovation, and drive ethical, sustainable change in complex environments.
Overview on State of the Art of Causal Inference
Several established approaches have been developed for causal inference, each with specific strengths and limitations depending on the complexity and type of data being analyzed. Hereโs an overview of some major methods:
1. Granger Causality (GC): Originally developed for linear time series data, GC tests whether one time series can forecast another. It has been expanded to handle nonlinear and multivariate data, but its performance declines with high nonlinearity or noise. GC is limited in its ability to handle complex causal structures, such as redundant or synergistic causality, and it typically assumes a parametric, linear relationship.
2. Conditional Transfer Entropy (CTE): An information-theoretic approach that quantifies causality by assessing how much information past values of one variable provide about the future values of another, conditioned on other variables. CTE is nonparametric and works well with nonlinear relationships, but it may struggle with redundancy (where multiple causes provide overlapping information) and may produce negative values, complicating interpretation.
3. Convergent Cross Mapping (CCM): CCM uses Takensโ embedding theorem to reconstruct attractor manifolds from time series data, allowing researchers to infer causal connections in complex, nonlinear systems. CCM is particularly useful for dynamic ecological or biological systems, but it can be sensitive to noise and may require extensive data for accurate results.
4. Peter-Clark Momentary Conditional Independence (PCMCI): This algorithm uses conditional independence tests to identify causal links and is effective at handling multivariate and nonlinear dependencies in time series data. PCMCI can be extended to capture contemporaneous (near-instantaneous) links, but it may struggle with high levels of redundant causality, which can lead to erroneous causal links if not carefully managed.
5. Directed Information (DI) and Transfer Entropy (TE): These methods are rooted in information theory and measure causality by assessing reductions in uncertainty (entropy) about one variable given knowledge of another. TE, a form of DI, can capture both linear and nonlinear relationships but, like GC, has limitations with redundant and synergistic effects.
6. SURD (Synergistic-Unique-Redundant Decomposition): Recently introduced, SURD addresses key limitations of traditional approaches by decomposing causality into unique, redundant, and synergistic components. It also provides a causality leak metric to account for influences from unobserved variables, offering a more comprehensive view of causality in systems with complex interdependencies.
Each approach has particular domains of applicationโe.g., GC and CTE in econometrics, PCMCI in neuroscience and climate science, and CCM in ecological studies. The SURD method builds on these by tackling specific gaps, such as the quantification of unobserved variables and the decomposition of causality types, enhancing our capacity to interpret complex causal systems.
Whatโs Left to Discover Relevant to Causal Inference SURD Developments?
The Synergistic-Unique-Redundant Decomposition (SURD) approach marks a significant advancement in causal inference, but there remain several frontier areas to explore, particularly as SURD opens up new avenues in understanding and quantifying causality. Here are some of the key areas left to explore:
1. Refinement of Causality Leak Analysis: SURD introduces the concept of causality leak, which quantifies the impact of unobserved variables.
2. Scaling SURD for High-Dimensional Systems: As data dimensions continue to grow, especially with the rise of big data and the Internet of Things, it will be essential to scale SURD efficiently.
3. Improving Robustness to Noise and Missing Data: While SURD is designed to be resilient to noise and incomplete data, further research could improve its robustness.
4. Integration with Machine Learning: Combining SURD with machine learning models could lead to more interpretable AI systems that are grounded in causal relationships.
5. Exploration of Real-Time Causal Inference: Real-time causal inference is an emerging area with potential applications in adaptive systems, autonomous vehicles, and smart infrastructure.
6. Extending Causal Inference to Nonlinear and Multi-Timescale Systems: SURDโs current framework addresses many nonlinear dependencies, but further development could make it even more applicable to highly nonlinear or multi-timescale systems, such as those found in climate science, neurobiology, and social dynamics.
7. Causal Inference in Counterfactual and Scenario Analysis: Causal inference is essential for evaluating โwhat ifโ scenarios, but traditional methods often face challenges with counterfactual predictions.
8. Applications in Ethical AI and Social Science: Causal inference is crucial for making ethically sound AI systems, particularly in areas like fairness, accountability, and transparency.
9. Development of User-Friendly Toolkits and Software: For SURD to become widely adopted, accessible tools and software implementations are necessary.
More details on the above, for the super curious.
1. Refinement of Causality Leak Analysis: SURD introduces the concept of causality leak, which quantifies the impact of unobserved variables. Future research could refine how we detect and interpret these leaks, especially in complex, high-dimensional systems. Enhancing causality leak metrics could help identify hidden variables that might be crucial to understanding systemic behaviors, such as unknown genetic factors in biology or unmeasured climate influences.
2. Scaling SURD for High-Dimensional Systems: As data dimensions continue to grow, especially with the rise of big data and the Internet of Things, it will be essential to scale SURD efficiently. Developing algorithms and computational tools that enable SURD to handle even larger datasets with minimal loss of precision would be a major step forward, making it practical for applications in areas like genomics, urban systems, and high-resolution climate modeling.
3. Improving Robustness to Noise and Missing Data: While SURD is designed to be resilient to noise and incomplete data, further research could improve its robustness. Enhanced techniques for handling stochasticity could make SURD even more effective in fields where data is inherently noisy, such as financial markets or environmental monitoring. Better handling of missing data within the causal framework would also expand its applicability.
4. Integration with Machine Learning: Combining SURD with machine learning models could lead to more interpretable AI systems that are grounded in causal relationships. This fusion could help machine learning algorithms not only predict outcomes but also understand underlying causes. Such integration would be especially valuable in healthcare, where interpretability and causality are crucial for personalized medicine and treatment planning.
5. Exploration of Real-Time Causal Inference: Real-time causal inference is an emerging area with potential applications in adaptive systems, autonomous vehicles, and smart infrastructure. Developing SURD-based methods that can infer causality in real time could help these systems make more responsive and informed decisions based on continuously incoming data.
6. Extending Causal Inference to Nonlinear and Multi-Timescale Systems: SURDโs current framework addresses many nonlinear dependencies, but further development could make it even more applicable to highly nonlinear or multi-timescale systems, such as those found in climate science, neurobiology, and social dynamics. Extending SURDโs capabilities here could deepen our understanding of causality in systems where effects are delayed or accumulate over time.
7. Causal Inference in Counterfactual and Scenario Analysis: Causal inference is essential for evaluating โwhat ifโ scenarios, but traditional methods often face challenges with counterfactual predictions. Extending SURD to handle counterfactual analysis in more complex scenarios could improve its utility in policy-making and risk assessment, such as modeling the long-term impacts of climate interventions or economic reforms.
8. Applications in Ethical AI and Social Science: Causal inference is crucial for making ethically sound AI systems, particularly in areas like fairness, accountability, and transparency. Expanding SURD to better address ethical considerationsโsuch as distinguishing between genuine causal relationships and spurious correlations that might reinforce biasโcould support the creation of socially responsible technologies.
9. Development of User-Friendly Toolkits and Software: For SURD to become widely adopted, accessible tools and software implementations are necessary. Developing these resources would enable practitioners in diverse fields, including social sciences, biology, and engineering, to use SURD without needing deep technical expertise, making causal inference a more common component in data analysis.
These frontier areas highlight both the promise and complexity of causal inference with SURD. Continued exploration could position SURD not just as a tool for causal analysis, but as a foundational approach for understanding causality across disciplines, ultimately leading to more robust, transparent, and impactful applications.
When youโre curious about how to apply SURD or other Causal Inference analytical approaches to your transformation projects, get in touch with the Prism14 team.
Thanks for reading and sharing!
by
Tags:
Leave a Reply